Session + Live Q&A
Differentiable Programming in Kotlin
Over the last few years, several frameworks have been developed to support differentiability. The most popular are PyTorch, TensorFlow and JAX which are all built on Python. These frameworks are oriented towards machine learning which involve building a model, performing batched computations on large tensors, and then using backpropagation to make incremental corrections to that model. These frameworks have been optimized for that purpose.
There are many other uses for differentiability which don't fit that paradigm as well. Physics simulations, computer graphics, and some novel machine learning techniques also need differentiability but have additional requirements. In particular, along with differentiability, fast bespoke calculations are often needed. This is difficult to do in Python whose performance is usually an order of magnitude slower than C or Java.
At Facebook, we are developing a new differentiable programming framework for Kotlin. While we provide a library to support typical machine learning use cases, we have taken an approach that is compiler-aware. As a result, we can detect tensor shape errors at compile time and also benefit from compile-time optimizations. We also support differentiability on sparse tensors which are gaining more popularity as the data being collected continues to grow at a staggering pace.
Speaker
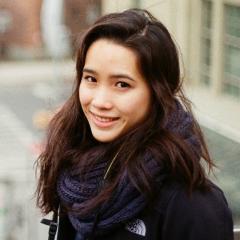
From the same track
Getting The Most Out Of Sandboxing
Wednesday May 19 / 11:10AM EDT
Privilege separation and reduction ("sandboxing") has significantly improved software security, and in many applications is a baseline requirement for safe design. (In fact, there are still many applications that can and should adopt sandboxing.)Although necessary, sandboxing is not...
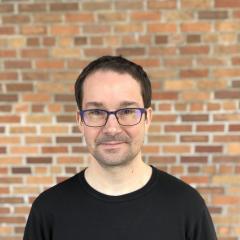
Chris Palmer
Software Security Engineer on Chrome @Google
Co-Designing Raft + Thread-per-Core Execution Model for the Kafka-API
Wednesday May 19 / 10:10AM EDT
Sometimes you get to reinvent the wheel when the road changes. Redpanda is a drop-in replacement for Apache Kafka®, designed from the ground up for modern hardware. Hardware looks nothing like it did 10 years ago. NVMe disks are 1000X faster than spinning disks. Cloud computers offer 30X more...
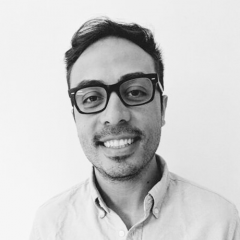
Alex Gallego
Founder and CEO @VectorizedIO
Panel: Future of Language Support for ML
Wednesday May 19 / 12:10PM EDT
In this panel, we'll take a look at the state of the art of ML/AI development and how advances in language technology (specifically differentiable programming languages) can help.
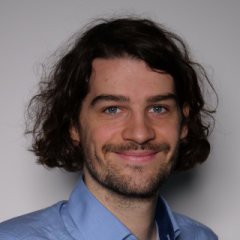
Jendrik Jördening
CTO @Nooxit
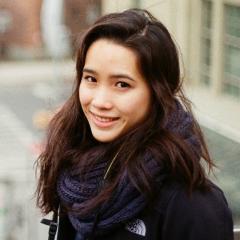
Irene Dea
Software Engineer @Facebook
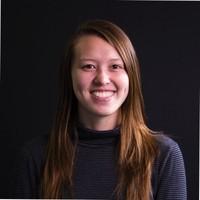
Alanna Tempest
Software Engineer @Facebook